Meet CBI Faculty Hosts: Virginia Smith and Steven Wu
Virginia Smith and Steven Wu
Aug 2, 2023
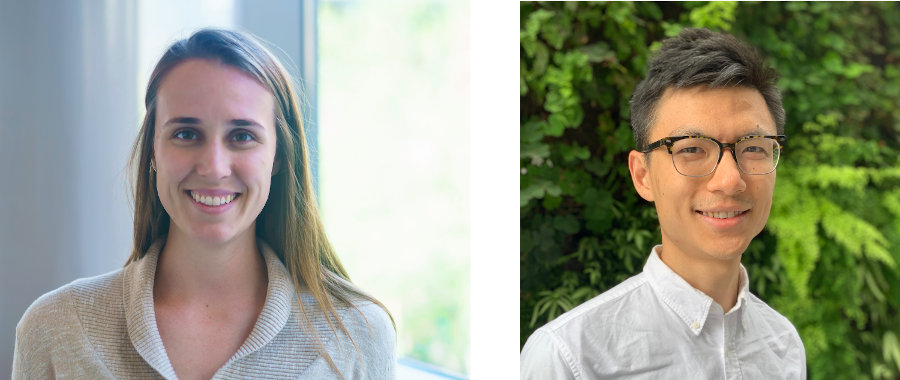
Over the past decade, the proliferation of machine learning coupled with the availability of large swaths of data have led to remarkable technological advances. However, the ubiquitous nature and increasing volume of data collection and processing has raised concerns about privacy infringement and increased the potential for data misuse. Enabling robust privacy measures to alleviate such concerns is essential for future adoption of such systems.
Our research cooperation focuses on the area of federated learning, which seeks to enable privacy-preserving machine learning across distributed data silos, such as networks of edge devices or collections of organizations. In this context, we look forward to the continued collaboration with Pratiksha Thaker as one of the recipients of the prestigious Carnegie Bosch Institute postdoctoral fellowship. Thaker graduated with her Ph.D. from Stanford, where she worked on approaches for distributed data processing systems. She aims to leverage this expertise in her postdoc to bridge the domains of distributed systems and privacy enhancing technologies and deliver practical, usable systems for private data analysis and machine learning.
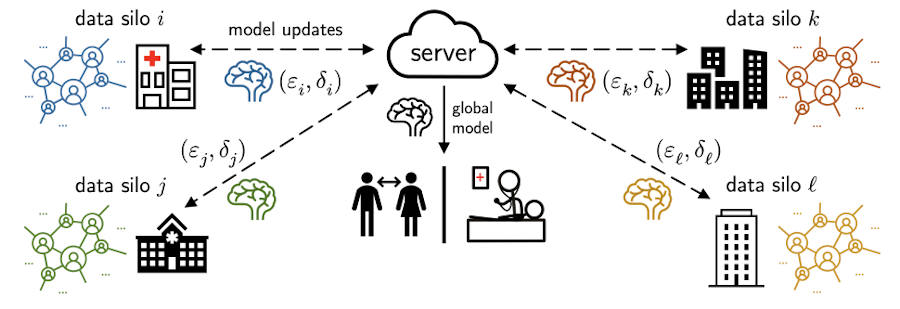
Example of a federated learning system
Despite significant research attention and a strong theoretical grounding, existing approaches for data privacy have seen limited use in real-world deployments of machine learning. The aim of our work will be to advance the frontiers of privacy-preserving machine learning with a focus on not only providing formal privacy guarantees but ensuring that the systems are also practical and deployable. We are excited to be working with Thaker towards this goal. Thaker has a collaborative mindset and unique skill set that are at the intersection of privacy, machine learning, and distributed systems, which makes her both uniquely poised to deliver on the next generation of practical, deployable systems, as well as a stellar representative of the CBI fellowship.
A standout feature of the Carnegie Bosch Fellowship is the expansive freedom and flexibility it affords its fellows, enabling them to pursue and address the most audacious and exhilarating research challenges through interdisciplinary research. Broad cooperation is necessary ensure the real-world impact of privacy-preserving machine learning research. Thaker has a track record of seeking and fostering successful collaborations across academia and industry. We strongly believe that this will enable her to utilize the full resources of the fellowship towards the success of this work.
The ultimate goal of our work is to make the process of learning over private, distributed data silos as seamless and secure as learning over centralized data. This fellowship will provide freedom to explore new, creative, interdisciplinary approaches that take us closer to this lofty goal. We are excited to be working with Thaker towards this.